Android Neural Networks API (NNAPI) — это API Android C, предназначенный для выполнения ресурсоемких операций машинного обучения на устройствах Android. NNAPI предназначен для обеспечения базового уровня функциональности для платформ машинного обучения более высокого уровня, таких как TensorFlow Lite и Caffe2, которые создают и обучают нейронные сети. API доступен на всех устройствах Android под управлением Android 8.1 (уровень API 27) или выше, но в Android 15 он устарел.
NNAPI поддерживает логический вывод, применяя данные с устройств Android к предварительно обученным моделям, определенным разработчиком. Примеры вывода включают классификацию изображений, прогнозирование поведения пользователя и выбор подходящих ответов на поисковый запрос.
Инференция на устройстве имеет множество преимуществ:
- Задержка : вам не нужно отправлять запрос через сетевое соединение и ждать ответа. Например, это может быть критично для видеоприложений, обрабатывающих последовательные кадры, поступающие с камеры.
- Доступность : приложение работает даже вне зоны покрытия сети.
- Скорость : новое оборудование, предназначенное для обработки нейронных сетей, обеспечивает значительно более быстрые вычисления, чем один только процессор общего назначения.
- Конфиденциальность : данные не покидают устройство Android.
- Стоимость : ферма серверов не требуется, если все вычисления выполняются на устройстве Android.
Есть также компромиссы, о которых следует помнить разработчику:
- Использование системы . Оценка нейронных сетей требует большого количества вычислений, что может увеличить расход заряда батареи. Вам следует рассмотреть возможность мониторинга состояния батареи, если это беспокоит ваше приложение, особенно при длительных вычислениях.
- Размер приложения : обратите внимание на размер ваших моделей. Модели могут занимать несколько мегабайт места. Если объединение больших моделей в APK может оказать чрезмерное влияние на ваших пользователей, вы можете рассмотреть возможность загрузки моделей после установки приложения, использования моделей меньшего размера или выполнения вычислений в облаке. NNAPI не предоставляет возможности запуска моделей в облаке.
См. образец API нейронных сетей Android , чтобы увидеть один пример использования NNAPI.
Понимание среды выполнения API нейронных сетей
NNAPI предназначен для вызова библиотеками, платформами и инструментами машинного обучения, которые позволяют разработчикам обучать свои модели за пределами устройства и развертывать их на устройствах Android. Приложения обычно не используют NNAPI напрямую, а вместо этого используют платформы машинного обучения более высокого уровня. Эти платформы, в свою очередь, могут использовать NNAPI для выполнения аппаратно-ускоренных операций вывода на поддерживаемых устройствах.
В зависимости от требований приложения и аппаратных возможностей устройства Android среда выполнения нейронной сети Android может эффективно распределять вычислительную нагрузку между доступными процессорами на устройстве, включая выделенное оборудование нейронной сети, графические процессоры (GPU) и процессоры цифровых сигналов (DSP).
Для устройств Android, у которых отсутствует специализированный драйвер поставщика, среда выполнения NNAPI выполняет запросы на ЦП.
На рисунке 1 показана системная архитектура высокого уровня для NNAPI.
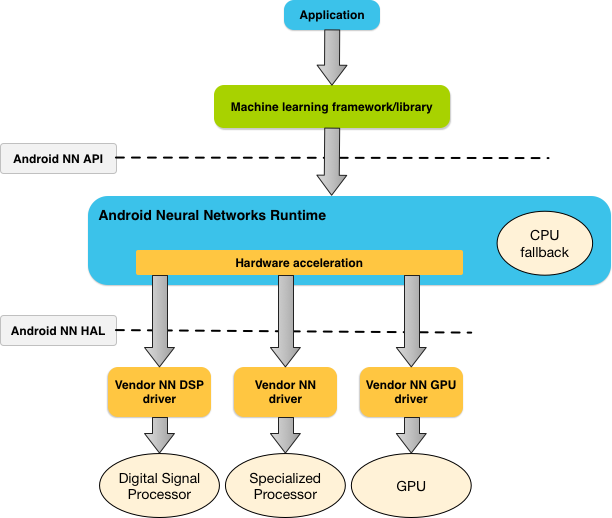
Модель программирования API нейронных сетей
Чтобы выполнять вычисления с использованием NNAPI, сначала необходимо построить ориентированный граф, определяющий выполняемые вычисления. Этот граф вычислений в сочетании с входными данными (например, весами и смещениями, переданными из среды машинного обучения) формирует модель для оценки времени выполнения NNAPI.
NNAPI использует четыре основные абстракции:
- Модель : граф вычислений математических операций и постоянных значений, полученных в процессе обучения. Эти операции специфичны для нейронных сетей. Они включают в себя двумерную (2D) свертку , логистическую ( сигмовидную ) активацию, выпрямленную линейную активацию (ReLU) и многое другое. Создание модели — синхронная операция. После успешного создания его можно повторно использовать в потоках и компиляциях. В NNAPI модель представлена как экземпляр
ANeuralNetworksModel
. - Компиляция : представляет конфигурацию для компиляции модели NNAPI в код нижнего уровня. Создание компиляции — синхронная операция. После успешного создания его можно повторно использовать в разных потоках и выполнениях. В NNAPI каждая компиляция представлена как экземпляр
ANeuralNetworksCompilation
. - Память : представляет общую память, файлы, отображаемые в памяти, и аналогичные буферы памяти. Использование буфера памяти позволяет среде выполнения NNAPI более эффективно передавать данные драйверам. Приложение обычно создает один буфер общей памяти, который содержит все тензоры, необходимые для определения модели. Вы также можете использовать буферы памяти для хранения входных и выходных данных экземпляра выполнения. В NNAPI каждый буфер памяти представлен как экземпляр
ANeuralNetworksMemory
. Выполнение : интерфейс для применения модели NNAPI к набору входных данных и сбора результатов. Выполнение может выполняться синхронно или асинхронно.
При асинхронном выполнении несколько потоков могут ожидать одного и того же выполнения. Когда это выполнение завершается, все потоки освобождаются.
В NNAPI каждое выполнение представлено как экземпляр
ANeuralNetworksExecution
.
На рис. 2 показан основной процесс программирования.
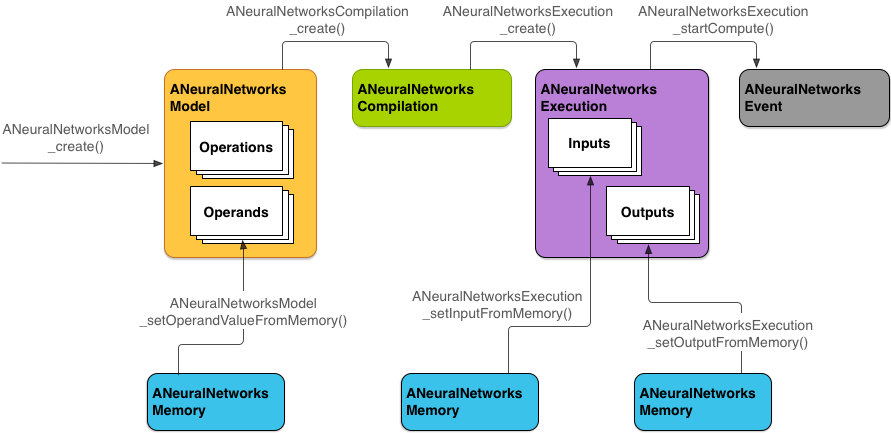
Оставшаяся часть этого раздела описывает шаги по настройке модели NNAPI для выполнения вычислений, компиляции модели и выполнения скомпилированной модели.
Предоставить доступ к данным обучения
Ваши обученные данные о весах и смещениях, скорее всего, хранятся в файле. Чтобы предоставить среде выполнения NNAPI эффективный доступ к этим данным, создайте экземпляр ANeuralNetworksMemory
, вызвав функцию ANeuralNetworksMemory_createFromFd()
и передав файловый дескриптор открытого файла данных. Вы также указываете флаги защиты памяти и смещение, где начинается область общей памяти в файле.
// Create a memory buffer from the file that contains the trained data
ANeuralNetworksMemory* mem1 = NULL;
int fd = open("training_data", O_RDONLY);
ANeuralNetworksMemory_createFromFd(file_size, PROT_READ, fd, 0, &mem1);
Хотя в этом примере мы используем только один экземпляр ANeuralNetworksMemory
для всех наших весов, можно использовать более одного экземпляра ANeuralNetworksMemory
для нескольких файлов.
Используйте собственные аппаратные буферы
Вы можете использовать собственные аппаратные буферы для входных и выходных данных модели, а также постоянных значений операндов. В некоторых случаях ускоритель NNAPI может получать доступ к объектам AHardwareBuffer
без необходимости копирования данных драйвером. AHardwareBuffer
имеет множество различных конфигураций, и не каждый ускоритель NNAPI может поддерживать все эти конфигурации. Из-за этого ограничения обратитесь к ограничениям, перечисленным в справочной документации ANeuralNetworksMemory_createFromAHardwareBuffer
, и заранее протестируйте на целевых устройствах, чтобы убедиться, что компиляции и выполнения, использующие AHardwareBuffer
, ведут себя должным образом, используя назначение устройств для указания ускорителя.
Чтобы разрешить среде выполнения NNAPI доступ к объекту AHardwareBuffer
, создайте экземпляр ANeuralNetworksMemory
, вызвав функцию ANeuralNetworksMemory_createFromAHardwareBuffer
и передав объект AHardwareBuffer
, как показано в следующем примере кода:
// Configure and create AHardwareBuffer object AHardwareBuffer_Desc desc = ... AHardwareBuffer* ahwb = nullptr; AHardwareBuffer_allocate(&desc, &ahwb); // Create ANeuralNetworksMemory from AHardwareBuffer ANeuralNetworksMemory* mem2 = NULL; ANeuralNetworksMemory_createFromAHardwareBuffer(ahwb, &mem2);
Когда NNAPI больше не требуется доступ к объекту AHardwareBuffer
, освободите соответствующий экземпляр ANeuralNetworksMemory
:
ANeuralNetworksMemory_free(mem2);
Примечание:
- Вы можете использовать
AHardwareBuffer
только для всего буфера; вы не можете использовать его с параметромARect
. - Среда выполнения NNAPI не очищает буфер. Прежде чем планировать выполнение, вам необходимо убедиться, что входной и выходной буферы доступны.
- Дескрипторы файлов ограждения синхронизации не поддерживаются.
- Для
AHardwareBuffer
с форматами и битами использования, зависящими от поставщика, реализация поставщика должна определить, кто отвечает за очистку кэша: клиент или драйвер.
Модель
Модель — это фундаментальная единица вычислений в NNAPI. Каждая модель определяется одним или несколькими операндами и операциями.
Операнды
Операнды — это объекты данных, используемые при определении графа. К ним относятся входные и выходные данные модели, промежуточные узлы, содержащие данные, передаваемые от одной операции к другой, и константы, передаваемые в эти операции.
В модели NNAPI можно добавлять два типа операндов: скаляры и тензоры .
Скаляр представляет одно значение. NNAPI поддерживает скалярные значения в логическом формате, 16-битном формате с плавающей запятой, 32-битном формате с плавающей запятой, 32-битном целочисленном формате и 32-битном целочисленном формате без знака.
Большинство операций в NNAPI используют тензоры. Тензоры — это n-мерные массивы. NNAPI поддерживает тензоры с 16-битными числами с плавающей запятой, 32-битными числами с плавающей запятой, 8-битными квантованными , 16-битными квантованными, 32-битными целыми числами и 8-битными логическими значениями.
Например, на рисунке 3 представлена модель с двумя операциями: сложением и последующим умножением. Модель принимает входной тензор и создает один выходной тензор.
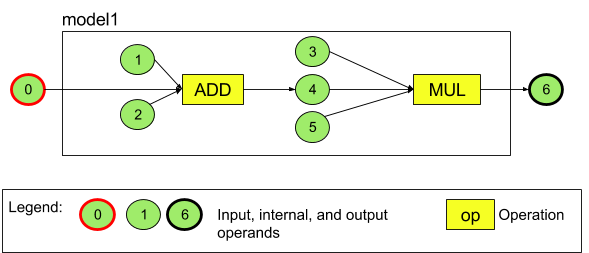
Модель выше имеет семь операндов. Эти операнды неявно идентифицируются по индексу порядка, в котором они добавляются в модель. Первый добавленный операнд имеет индекс 0, второй — индекс 1 и так далее. Операнды 1, 2, 3 и 5 являются постоянными операндами.
Порядок добавления операндов не имеет значения. Например, выходной операнд модели может быть добавлен первым. Важной частью является использование правильного значения индекса при обращении к операнду.
Операнды имеют типы. Они указываются при добавлении в модель.
Операнд не может использоваться одновременно как вход и выход модели.
Каждый операнд должен быть либо входным параметром модели, либо константой, либо выходным операндом ровно одной операции.
Дополнительную информацию об использовании операндов см. в разделе «Подробнее об операндах» .
Операции
Операция определяет вычисления, которые необходимо выполнить. Каждая операция состоит из следующих элементов:
- тип операции (например, сложение, умножение, свертка),
- список индексов операндов, которые операция использует для ввода, и
- список индексов операндов, которые операция использует для вывода.
Порядок в этих списках имеет значение; ожидаемые входные и выходные данные для каждого типа операции см. в справочнике по API NNAPI .
Перед добавлением операции необходимо добавить в модель операнды, которые операция использует или создает.
Порядок добавления операций не имеет значения. NNAPI опирается на зависимости, установленные графом вычислений операндов и операций, для определения порядка выполнения операций.
Операции, которые поддерживает NNAPI, приведены в таблице ниже:
Известная проблема на уровне API 28: при передаче тензоров ANEURALNETWORKS_TENSOR_QUANT8_ASYMM
в операцию ANEURALNETWORKS_PAD
, которая доступна на Android 9 (уровень API 28) и более поздних версиях, выходные данные NNAPI могут не соответствовать выводам платформ машинного обучения более высокого уровня, таких как TensorFlow Lite . Вместо этого вам следует передать только ANEURALNETWORKS_TENSOR_FLOAT32
. Проблема решена в Android 10 (уровень API 29) и выше.
Сборка моделей
В следующем примере мы создаем модель с двумя операциями, показанную на рисунке 3 .
Чтобы построить модель, выполните следующие действия:
Вызовите функцию
ANeuralNetworksModel_create()
, чтобы определить пустую модель.ANeuralNetworksModel* model = NULL; ANeuralNetworksModel_create(&model);
Добавьте операнды в свою модель, вызвав
ANeuralNetworks_addOperand()
. Их типы данных определяются с использованием структуры данныхANeuralNetworksOperandType
.// In our example, all our tensors are matrices of dimension [3][4] ANeuralNetworksOperandType tensor3x4Type; tensor3x4Type.type = ANEURALNETWORKS_TENSOR_FLOAT32; tensor3x4Type.scale = 0.f; // These fields are used for quantized tensors tensor3x4Type.zeroPoint = 0; // These fields are used for quantized tensors tensor3x4Type.dimensionCount = 2; uint32_t dims[2] = {3, 4}; tensor3x4Type.dimensions = dims;
// We also specify operands that are activation function specifiers ANeuralNetworksOperandType activationType; activationType.type = ANEURALNETWORKS_INT32; activationType.scale = 0.f; activationType.zeroPoint = 0; activationType.dimensionCount = 0; activationType.dimensions = NULL;
// Now we add the seven operands, in the same order defined in the diagram ANeuralNetworksModel_addOperand(model, &tensor3x4Type); // operand 0 ANeuralNetworksModel_addOperand(model, &tensor3x4Type); // operand 1 ANeuralNetworksModel_addOperand(model, &activationType); // operand 2 ANeuralNetworksModel_addOperand(model, &tensor3x4Type); // operand 3 ANeuralNetworksModel_addOperand(model, &tensor3x4Type); // operand 4 ANeuralNetworksModel_addOperand(model, &activationType); // operand 5 ANeuralNetworksModel_addOperand(model, &tensor3x4Type); // operand 6Для операндов, имеющих постоянные значения, таких как веса и смещения, которые ваше приложение получает в процессе обучения, используйте функции
ANeuralNetworksModel_setOperandValue()
иANeuralNetworksModel_setOperandValueFromMemory()
.В следующем примере мы устанавливаем постоянные значения из файла обучающих данных, соответствующих буферу памяти, который мы создали в разделе «Предоставление доступа к обучающим данным» .
// In our example, operands 1 and 3 are constant tensors whose values were // established during the training process const int sizeOfTensor = 3 * 4 * 4; // The formula for size calculation is dim0 * dim1 * elementSize ANeuralNetworksModel_setOperandValueFromMemory(model, 1, mem1, 0, sizeOfTensor); ANeuralNetworksModel_setOperandValueFromMemory(model, 3, mem1, sizeOfTensor, sizeOfTensor);
// We set the values of the activation operands, in our example operands 2 and 5 int32_t noneValue = ANEURALNETWORKS_FUSED_NONE; ANeuralNetworksModel_setOperandValue(model, 2, &noneValue, sizeof(noneValue)); ANeuralNetworksModel_setOperandValue(model, 5, &noneValue, sizeof(noneValue));Для каждой операции в ориентированном графе, которую вы хотите вычислить, добавьте операцию в свою модель, вызвав функцию
ANeuralNetworksModel_addOperation()
.В качестве параметров этого вызова ваше приложение должно предоставить:
- тип операции
- количество входных значений
- массив индексов для входных операндов
- количество выходных значений
- массив индексов для выходных операндов
Обратите внимание, что операнд не может использоваться одновременно для ввода и вывода одной и той же операции.
// We have two operations in our example // The first consumes operands 1, 0, 2, and produces operand 4 uint32_t addInputIndexes[3] = {1, 0, 2}; uint32_t addOutputIndexes[1] = {4}; ANeuralNetworksModel_addOperation(model, ANEURALNETWORKS_ADD, 3, addInputIndexes, 1, addOutputIndexes);
// The second consumes operands 3, 4, 5, and produces operand 6 uint32_t multInputIndexes[3] = {3, 4, 5}; uint32_t multOutputIndexes[1] = {6}; ANeuralNetworksModel_addOperation(model, ANEURALNETWORKS_MUL, 3, multInputIndexes, 1, multOutputIndexes);Определите, какие операнды модель должна рассматривать как входные и выходные данные, вызвав функцию
ANeuralNetworksModel_identifyInputsAndOutputs()
.// Our model has one input (0) and one output (6) uint32_t modelInputIndexes[1] = {0}; uint32_t modelOutputIndexes[1] = {6}; ANeuralNetworksModel_identifyInputsAndOutputs(model, 1, modelInputIndexes, 1 modelOutputIndexes);
При необходимости укажите, разрешено ли вычислять
ANEURALNETWORKS_TENSOR_FLOAT32
с таким же низким диапазоном или точностью, как у 16-битного формата с плавающей запятой IEEE 754, вызвавANeuralNetworksModel_relaxComputationFloat32toFloat16()
.Вызовите
ANeuralNetworksModel_finish()
чтобы завершить определение вашей модели. Если ошибок нет, эта функция возвращает код результатаANEURALNETWORKS_NO_ERROR
.ANeuralNetworksModel_finish(model);
Создав модель, вы можете компилировать ее любое количество раз и выполнять каждую компиляцию любое количество раз.
Поток управления
Чтобы включить поток управления в модель NNAPI, выполните следующие действия:
Создайте соответствующие подграфы выполнения (подграфы
then
иelse
для оператораIF
, подграфыcondition
иbody
для циклаWHILE
) как автономные моделиANeuralNetworksModel*
:ANeuralNetworksModel* thenModel = makeThenModel(); ANeuralNetworksModel* elseModel = makeElseModel();
Создайте операнды, которые ссылаются на эти модели в модели, содержащей поток управления:
ANeuralNetworksOperandType modelType = { .type = ANEURALNETWORKS_MODEL, }; ANeuralNetworksModel_addOperand(model, &modelType); // kThenOperandIndex ANeuralNetworksModel_addOperand(model, &modelType); // kElseOperandIndex ANeuralNetworksModel_setOperandValueFromModel(model, kThenOperandIndex, &thenModel); ANeuralNetworksModel_setOperandValueFromModel(model, kElseOperandIndex, &elseModel);
Добавьте операцию потока управления:
uint32_t inputs[] = {kConditionOperandIndex, kThenOperandIndex, kElseOperandIndex, kInput1, kInput2, kInput3}; uint32_t outputs[] = {kOutput1, kOutput2}; ANeuralNetworksModel_addOperation(model, ANEURALNETWORKS_IF, std::size(inputs), inputs, std::size(output), outputs);
Сборник
Шаг компиляции определяет, на каких процессорах будет выполняться ваша модель, и запрашивает соответствующие драйверы подготовиться к ее выполнению. Это может включать в себя генерацию машинного кода, специфичного для процессоров, на которых будет работать ваша модель.
Чтобы скомпилировать модель, выполните следующие действия:
Вызовите функцию
ANeuralNetworksCompilation_create()
, чтобы создать новый экземпляр компиляции.// Compile the model ANeuralNetworksCompilation* compilation; ANeuralNetworksCompilation_create(model, &compilation);
При желании вы можете использовать назначение устройств , чтобы явно выбрать, на каких устройствах выполнять выполнение.
При желании вы можете повлиять на то, как время выполнения будет зависеть от использования энергии аккумулятора и скорости выполнения. Вы можете сделать это, вызвав
ANeuralNetworksCompilation_setPreference()
.// Ask to optimize for low power consumption ANeuralNetworksCompilation_setPreference(compilation, ANEURALNETWORKS_PREFER_LOW_POWER);
Предпочтения, которые вы можете указать, включают в себя:
-
ANEURALNETWORKS_PREFER_LOW_POWER
: предпочитаете выполнение таким образом, чтобы минимизировать разряд батареи. Это желательно для часто выполняемых компиляций. -
ANEURALNETWORKS_PREFER_FAST_SINGLE_ANSWER
: предпочитать возвращать один ответ как можно быстрее, даже если это приводит к большему энергопотреблению. Это значение по умолчанию. -
ANEURALNETWORKS_PREFER_SUSTAINED_SPEED
: предпочитает максимизировать пропускную способность последовательных кадров, например, при обработке последовательных кадров, поступающих с камеры.
-
При желании вы можете настроить кэширование компиляции, вызвав
ANeuralNetworksCompilation_setCaching
.// Set up compilation caching ANeuralNetworksCompilation_setCaching(compilation, cacheDir, token);
Используйте
getCodeCacheDir()
дляcacheDir
. Указанныйtoken
должен быть уникальным для каждой модели в приложении.Завершите определение компиляции, вызвав
ANeuralNetworksCompilation_finish()
. Если ошибок нет, эта функция возвращает код результатаANEURALNETWORKS_NO_ERROR
.ANeuralNetworksCompilation_finish(compilation);
Обнаружение и назначение устройств
На устройствах Android под управлением Android 10 (уровень API 29) и выше NNAPI предоставляет функции, которые позволяют библиотекам и приложениям платформы машинного обучения получать информацию о доступных устройствах и указывать устройства, которые будут использоваться для выполнения. Предоставление информации о доступных устройствах позволяет приложениям получать точную версию драйверов, найденных на устройстве, чтобы избежать известных несовместимостей. Предоставляя приложениям возможность указывать, какие устройства должны выполнять различные разделы модели, приложения можно оптимизировать для устройства Android, на котором они развернуты.
Обнаружение устройств
Используйте ANeuralNetworks_getDeviceCount
, чтобы получить количество доступных устройств. Для каждого устройства используйте ANeuralNetworks_getDevice
, чтобы установить для экземпляра ANeuralNetworksDevice
ссылку на это устройство.
Получив ссылку на устройство, вы можете получить дополнительную информацию об этом устройстве, используя следующие функции:
-
ANeuralNetworksDevice_getFeatureLevel
-
ANeuralNetworksDevice_getName
-
ANeuralNetworksDevice_getType
-
ANeuralNetworksDevice_getVersion
Назначение устройства
Используйте ANeuralNetworksModel_getSupportedOperationsForDevices
, чтобы определить, какие операции модели можно запускать на определенных устройствах.
Чтобы контролировать, какие ускорители использовать для выполнения, вызовите ANeuralNetworksCompilation_createForDevices
вместо ANeuralNetworksCompilation_create
. Используйте полученный объект ANeuralNetworksCompilation
как обычно. Функция возвращает ошибку, если предоставленная модель содержит операции, которые не поддерживаются выбранными устройствами.
Если указано несколько устройств, среда выполнения отвечает за распределение работы между устройствами.
Подобно другим устройствам, реализация ЦП NNAPI представлена ANeuralNetworksDevice
с именем nnapi-reference
и типом ANEURALNETWORKS_DEVICE_TYPE_CPU
. При вызове ANeuralNetworksCompilation_createForDevices
реализация ЦП не используется для обработки случаев сбоя при компиляции и выполнении модели.
В обязанности приложения входит разделение модели на подмодели, которые могут работать на указанных устройствах. Приложения, которым не требуется выполнять секционирование вручную, должны продолжать вызывать более простой метод ANeuralNetworksCompilation_create
, чтобы использовать все доступные устройства (включая ЦП) для ускорения модели. Если модель не может быть полностью поддержана устройствами, указанными вами с помощью ANeuralNetworksCompilation_createForDevices
, возвращается ANEURALNETWORKS_BAD_DATA
.
Разделение модели
Если для модели доступно несколько устройств, среда выполнения NNAPI распределяет работу между устройствами. Например, если ANeuralNetworksCompilation_createForDevices
было предоставлено более одного устройства, все указанные будут учитываться при распределении работы. Обратите внимание: если устройство ЦП отсутствует в списке, выполнение ЦП будет отключено. При использовании ANeuralNetworksCompilation_create
будут учитываться все доступные устройства, включая ЦП.
Распределение осуществляется путем выбора из списка доступных устройств для каждой операции в модели устройства, поддерживающего операцию и декларирующего лучшую производительность, т. е. самое быстрое время выполнения или самое низкое энергопотребление, в зависимости от предпочтений выполнения, указанных клиентом. Этот алгоритм разделения не учитывает возможную неэффективность, вызванную вводом-выводом между различными процессорами, поэтому при указании нескольких процессоров (либо явно при использовании ANeuralNetworksCompilation_createForDevices
, либо неявно при использовании ANeuralNetworksCompilation_create
) важно профилировать полученное приложение.
Чтобы понять, как ваша модель была разделена NNAPI, проверьте журналы Android на наличие сообщения (на уровне INFO с тегом ExecutionPlan
):
ModelBuilder::findBestDeviceForEachOperation(op-name): device-index
op-name
— это описательное имя операции в графе, а device-index
— это индекс устройства-кандидата в списке устройств. Этот список является входными данными для ANeuralNetworksCompilation_createForDevices
или, если используется ANeuralNetworksCompilation_createForDevices
, списком устройств, возвращаемым при переборе всех устройств с использованием ANeuralNetworks_getDeviceCount
и ANeuralNetworks_getDevice
.
Сообщение (на уровне INFO с тегом ExecutionPlan
):
ModelBuilder::partitionTheWork: only one best device: device-name
Это сообщение указывает на то, что весь граф был ускорен на device-name
.
Исполнение
На этапе выполнения модель применяется к набору входных данных, а выходные данные вычислений сохраняются в одном или нескольких пользовательских буферах или областях памяти, выделенных вашим приложением.
Чтобы выполнить скомпилированную модель, выполните следующие действия:
Вызовите функцию
ANeuralNetworksExecution_create()
, чтобы создать новый экземпляр выполнения.// Run the compiled model against a set of inputs ANeuralNetworksExecution* run1 = NULL; ANeuralNetworksExecution_create(compilation, &run1);
Укажите, где ваше приложение считывает входные значения для вычислений. Ваше приложение может считывать входные значения либо из пользовательского буфера, либо из выделенного пространства памяти, вызывая
ANeuralNetworksExecution_setInput()
илиANeuralNetworksExecution_setInputFromMemory()
соответственно.// Set the single input to our sample model. Since it is small, we won't use a memory buffer float32 myInput[3][4] = { ...the data... }; ANeuralNetworksExecution_setInput(run1, 0, NULL, myInput, sizeof(myInput));
Укажите, куда ваше приложение записывает выходные значения. Ваше приложение может записывать выходные значения либо в пользовательский буфер, либо в выделенное пространство памяти, вызывая
ANeuralNetworksExecution_setOutput()
илиANeuralNetworksExecution_setOutputFromMemory()
соответственно.// Set the output float32 myOutput[3][4]; ANeuralNetworksExecution_setOutput(run1, 0, NULL, myOutput, sizeof(myOutput));
Запланируйте запуск выполнения, вызвав функцию
ANeuralNetworksExecution_startCompute()
. Если ошибок нет, эта функция возвращает код результатаANEURALNETWORKS_NO_ERROR
.// Starts the work. The work proceeds asynchronously ANeuralNetworksEvent* run1_end = NULL; ANeuralNetworksExecution_startCompute(run1, &run1_end);
Вызовите функцию
ANeuralNetworksEvent_wait()
, чтобы дождаться завершения выполнения. Если выполнение прошло успешно, эта функция возвращает код результатаANEURALNETWORKS_NO_ERROR
. Ожидание может выполняться в другом потоке, отличном от того, который запускает выполнение.// For our example, we have no other work to do and will just wait for the completion ANeuralNetworksEvent_wait(run1_end); ANeuralNetworksEvent_free(run1_end); ANeuralNetworksExecution_free(run1);
При желании вы можете применить другой набор входных данных к скомпилированной модели, используя тот же экземпляр компиляции для создания нового экземпляра
ANeuralNetworksExecution
.// Apply the compiled model to a different set of inputs ANeuralNetworksExecution* run2; ANeuralNetworksExecution_create(compilation, &run2); ANeuralNetworksExecution_setInput(run2, ...); ANeuralNetworksExecution_setOutput(run2, ...); ANeuralNetworksEvent* run2_end = NULL; ANeuralNetworksExecution_startCompute(run2, &run2_end); ANeuralNetworksEvent_wait(run2_end); ANeuralNetworksEvent_free(run2_end); ANeuralNetworksExecution_free(run2);
Синхронное выполнение
Асинхронное выполнение тратит время на создание и синхронизацию потоков. Кроме того, задержка может сильно варьироваться: самые длинные задержки достигают 500 микросекунд между моментом уведомления или пробуждения потока и моментом его окончательной привязки к ядру ЦП.
Чтобы уменьшить задержку, вы можете вместо этого указать приложению выполнить синхронный вызов вывода во время выполнения. Этот вызов вернется только после завершения вывода, а не после его запуска. Вместо вызова ANeuralNetworksExecution_startCompute
для асинхронного вызова среды выполнения приложение вызывает ANeuralNetworksExecution_compute
для синхронного вызова среды выполнения. Вызов ANeuralNetworksExecution_compute
не принимает ANeuralNetworksEvent
и не связан с вызовом ANeuralNetworksEvent_wait
.
Пакетные казни
На устройствах Android под управлением Android 10 (уровень API 29) и выше NNAPI поддерживает пакетное выполнение через объект ANeuralNetworksBurst
. Пакетные исполнения — это последовательность выполнения одной и той же компиляции, которые происходят в быстрой последовательности, например, при работе с кадрами, снятыми с камеры, или с последовательными аудиосэмплами. Использование объектов ANeuralNetworksBurst
может привести к более быстрому выполнению, поскольку они указывают ускорителям, что ресурсы могут повторно использоваться между выполнениями и что ускорители должны оставаться в состоянии высокой производительности на протяжении всего пакета.
ANeuralNetworksBurst
вносит лишь небольшое изменение в обычный путь выполнения. Вы создаете пакетный объект с помощью ANeuralNetworksBurst_create
, как показано в следующем фрагменте кода:
// Create burst object to be reused across a sequence of executions ANeuralNetworksBurst* burst = NULL; ANeuralNetworksBurst_create(compilation, &burst);
Пакетные исполнения являются синхронными. Однако вместо использования ANeuralNetworksExecution_compute
для выполнения каждого вывода вы соединяете различные объекты ANeuralNetworksExecution
с одним и тем же ANeuralNetworksBurst
в вызовах функции ANeuralNetworksExecution_burstCompute
.
// Create and configure first execution object // ... // Execute using the burst object ANeuralNetworksExecution_burstCompute(execution1, burst); // Use results of first execution and free the execution object // ... // Create and configure second execution object // ... // Execute using the same burst object ANeuralNetworksExecution_burstCompute(execution2, burst); // Use results of second execution and free the execution object // ...
Освободите объект ANeuralNetworksBurst
с помощью ANeuralNetworksBurst_free
, когда он больше не нужен.
// Cleanup ANeuralNetworksBurst_free(burst);
Асинхронные очереди команд и изолированное выполнение
В Android 11 и более поздних версиях NNAPI поддерживает дополнительный способ планирования асинхронного выполнения с помощью метода ANeuralNetworksExecution_startComputeWithDependencies()
. При использовании этого метода выполнение ожидает сигнала обо всех зависимых событиях, прежде чем начать оценку. Как только выполнение завершено и выходные данные готовы к использованию, возвращается сигнал о возвращенном событии.
В зависимости от того, какие устройства обрабатывают выполнение, событие может поддерживаться барьером синхронизации . Вы должны вызвать ANeuralNetworksEvent_wait()
чтобы дождаться события и восстановить ресурсы, использованные при выполнении. Вы можете импортировать границы синхронизации в объект события с помощью ANeuralNetworksEvent_createFromSyncFenceFd()
и экспортировать границы синхронизации из объекта события с помощью ANeuralNetworksEvent_getSyncFenceFd()
.
Выходные данные с динамическим размером
Для поддержки моделей, в которых размер выходных данных зависит от входных данных — то есть, где размер не может быть определен во время выполнения модели — используйте ANeuralNetworksExecution_getOutputOperandRank
и ANeuralNetworksExecution_getOutputOperandDimensions
.
В следующем примере кода показано, как это сделать:
// Get the rank of the output uint32_t myOutputRank = 0; ANeuralNetworksExecution_getOutputOperandRank(run1, 0, &myOutputRank); // Get the dimensions of the output std::vector<uint32_t> myOutputDimensions(myOutputRank); ANeuralNetworksExecution_getOutputOperandDimensions(run1, 0, myOutputDimensions.data());
Очистка
Шаг очистки обеспечивает освобождение внутренних ресурсов, используемых для вычислений.
// Cleanup ANeuralNetworksCompilation_free(compilation); ANeuralNetworksModel_free(model); ANeuralNetworksMemory_free(mem1);
Управление ошибками и резервное использование ЦП
Если во время разделения возникает ошибка, если драйвер не может скомпилировать (часть модели) или если драйвер не может выполнить скомпилированную (часть модели) модель, NNAPI может вернуться к собственной реализации ЦП одной или нескольких операций.
Если клиент NNAPI содержит оптимизированные версии операции (как, например, TFLite), может быть полезно отключить резервный процессор ЦП и обрабатывать сбои с помощью оптимизированной реализации операции клиента.
В Android 10, если компиляция выполняется с использованием ANeuralNetworksCompilation_createForDevices
, резервное использование ЦП будет отключено.
В Android P выполнение NNAPI возвращается к ЦП, если выполнение драйвера завершается сбоем. Это также верно для Android 10, когда используется ANeuralNetworksCompilation_create
, а не ANeuralNetworksCompilation_createForDevices
.
Первое выполнение возвращается к этому единственному разделу, и если это по-прежнему не удается, оно повторяет всю модель на ЦП.
Если секционирование или компиляция не удались, вся модель будет опробована на ЦП.
Бывают случаи, когда некоторые операции не поддерживаются процессором, и в таких ситуациях компиляция или выполнение завершаются сбоем, а не откатываются назад.
Даже после отключения резервного использования ЦП в модели все еще могут быть операции, запланированные на ЦП. Если ЦП находится в списке процессоров, предоставленном ANeuralNetworksCompilation_createForDevices
, и является либо единственным процессором, поддерживающим эти операции, либо процессором, который заявляет о наилучшей производительности для этих операций, он будет выбран в качестве основного (без резервного) исполнителя.
Чтобы гарантировать отсутствие выполнения ЦП, используйте ANeuralNetworksCompilation_createForDevices
, исключив nnapi-reference
из списка устройств. Начиная с Android P, можно отключить резервный вариант во время выполнения сборок DEBUG, установив для свойства debug.nn.partition
значение 2.
Домены памяти
В Android 11 и более поздних версиях NNAPI поддерживает домены памяти, которые предоставляют интерфейсы распределителя для непрозрачных воспоминаний. Это позволяет приложениям передавать собственную память устройства при выполнении, так что NNAPI не копирует и не преобразовывает данные без необходимости при выполнении последовательных исполнений на одном и том же драйвере.
Функция домена памяти предназначена для тензоров, которые в основном являются внутренними по отношению к драйверу и не требуют частого доступа к стороне клиента. Примеры таких тензоров включают тензоры состояния в моделях последовательностей. Для тензоров, которым требуется частый доступ к ЦП на стороне клиента, вместо этого используйте пулы общей памяти.
Чтобы выделить непрозрачную память, выполните следующие действия:
Вызовите функцию
ANeuralNetworksMemoryDesc_create()
, чтобы создать новый дескриптор памяти:// Create a memory descriptor ANeuralNetworksMemoryDesc* desc; ANeuralNetworksMemoryDesc_create(&desc);
Укажите все предполагаемые роли ввода и вывода, вызвав
ANeuralNetworksMemoryDesc_addInputRole()
иANeuralNetworksMemoryDesc_addOutputRole()
.// Specify that the memory may be used as the first input and the first output // of the compilation ANeuralNetworksMemoryDesc_addInputRole(desc, compilation, 0, 1.0f); ANeuralNetworksMemoryDesc_addOutputRole(desc, compilation, 0, 1.0f);
При необходимости укажите размеры памяти, вызвав
ANeuralNetworksMemoryDesc_setDimensions()
.// Specify the memory dimensions uint32_t dims[] = {3, 4}; ANeuralNetworksMemoryDesc_setDimensions(desc, 2, dims);
Завершите определение дескриптора, вызвав
ANeuralNetworksMemoryDesc_finish()
.ANeuralNetworksMemoryDesc_finish(desc);
Выделите столько памяти, сколько вам нужно, передав дескриптор в
ANeuralNetworksMemory_createFromDesc()
.// Allocate two opaque memories with the descriptor ANeuralNetworksMemory* opaqueMem; ANeuralNetworksMemory_createFromDesc(desc, &opaqueMem);
Освободите дескриптор памяти, когда он вам больше не нужен.
ANeuralNetworksMemoryDesc_free(desc);
Клиент может использовать созданный объект ANeuralNetworksMemory
только с ANeuralNetworksExecution_setInputFromMemory()
или ANeuralNetworksExecution_setOutputFromMemory()
в соответствии с ролями, указанными в объекте ANeuralNetworksMemoryDesc
. Аргументы смещения и длины должны быть установлены в 0, что указывает на то, что используется вся память. Клиент также может явно устанавливать или извлекать содержимое памяти с помощью ANeuralNetworksMemory_copy()
.
Вы можете создавать непрозрачные воспоминания с ролями неопределенного размера или ранга. В этом случае создание памяти может завершиться неудачей со статусом ANEURALNETWORKS_OP_FAILED
, если оно не поддерживается базовым драйвером. Клиенту рекомендуется реализовать резервную логику, выделив достаточно большой буфер, поддерживаемый Ashmem или AHardwareBuffer
в режиме BLOB.
Когда NNAPI больше не требуется доступ к непрозрачному объекту памяти, освободите соответствующий экземпляр ANeuralNetworksMemory
:
ANeuralNetworksMemory_free(opaqueMem);
Измерение производительности
Вы можете оценить производительность своего приложения, измеряя время выполнения или профилируя.
Время выполнения
Если вы хотите определить общее время выполнения во время выполнения, вы можете использовать API синхронного выполнения и измерить время, затраченное на вызов. Если вы хотите определить общее время выполнения на более низком уровне программного стека, вы можете использовать ANeuralNetworksExecution_setMeasureTiming
и ANeuralNetworksExecution_getDuration
, чтобы получить:
- время выполнения на ускорителе (а не в драйвере, работающем на хост-процессоре).
- время выполнения в драйвере, включая время на акселераторе.
Время выполнения в драйвере исключает накладные расходы, например, на саму среду выполнения и IPC, необходимые для связи среды выполнения с драйвером.
Эти API измеряют продолжительность между событиями отправки и завершения работы, а не время, которое драйвер или ускоритель тратит на выполнение вывода, возможно, прерываемого переключением контекста.
Например, если начинается вывод 1, затем водитель прекращает работу для выполнения вывода 2, затем возобновляет работу и завершает вывод 1, то во время выполнения вывода 1 будет включено время, когда работа была остановлена для выполнения вывода 2.
Эта информация о времени может быть полезна для производственного развертывания приложения для сбора телеметрии для автономного использования. Вы можете использовать данные синхронизации, чтобы изменить приложение для повышения производительности.
При использовании этой функции имейте в виду следующее:
- Сбор информации о времени может привести к снижению производительности.
- Только драйвер способен подсчитать время, проведенное в себе или на ускорителе, не считая времени, проведенного в среде выполнения NNAPI и в IPC.
- Эти API можно использовать только с
ANeuralNetworksExecution
, созданным с помощьюANeuralNetworksCompilation_createForDevices
сnumDevices = 1
. - Ни один водитель не обязан иметь возможность сообщать информацию о времени.
Профилируйте свое приложение с помощью Android Systrace
Начиная с Android 10, NNAPI автоматически генерирует события SYSTRACE , которые вы можете использовать для предоставления вашего приложения.
Источник NNAPI поставляется с утилитой parse_systrace
для обработки событий Systrace, сгенерированных вашим приложением, и создания вида таблицы, показывающего время, проведенное на различных этапах жизненного цикла модели (инстанция, подготовка, выполнение компиляции и прекращение) и различные слои применения. Слои, в которых разделено ваше приложение:
-
Application
: основной код приложения -
Runtime
: NNAPI Runtime -
IPC
: взаимосвязь между процессом между временем выполнения NNAPI и кодом драйвера -
Driver
: процесс драйвера ускорителя.
Генерировать данные профилирования анализа
Предполагая, что вы проверили дерево источника AOSP в $ android_build_top, и, используя пример классификации Tflite Image в качестве целевого приложения, вы можете генерировать данные профилирования NNAPI со следующими шагами:
- Начните Systrace Android со следующей командой:
$ANDROID_BUILD_TOP/external/chromium-trace/systrace.py -o trace.html -a org.tensorflow.lite.examples.classification nnapi hal freq sched idle load binder_driver
Параметр -o trace.html
указывает, что следы будут записаны в trace.html
. При профилировании собственного приложения вам нужно будет заменить org.tensorflow.lite.examples.classification
с помощью имени процесса, указанного в манифесте вашего приложения.
Это будет занять одну из вашей консоли оболочки, не запускайте команду в фоновом режиме, так как он интерактивно ожидает завершения enter
.
- После того, как коллекционер Systrace запустит, запустите приложение и запустите тест на эталон.
В нашем случае вы можете запустить приложение классификации изображений из Android Studio или непосредственно с вашего пользовательского интерфейса Test Phone, если приложение уже установлено. Чтобы сгенерировать некоторые данные NNAPI, вам необходимо настроить приложение для использования NNAPI, выбрав NNAPI в качестве целевого устройства в диалоговом окне «Конфигурация приложения».
Когда тест завершается, прекратите Systrace, нажав
enter
на консольном терминале, активном с шага 1.Запустите утилиту
systrace_parser
Generate Совокупную статистику:
$ANDROID_BUILD_TOP/frameworks/ml/nn/tools/systrace_parser/parse_systrace.py --total-times trace.html
Паризер принимает следующие параметры: - --total-times
: показывает общее время, проведенное в слое, включая время, потраченное на ожидание исполнения при вызове базового уровня - - --print-detail
: печатает все события, которые были собраны из SYSTRACE - --per-execution
--json
Печать только в исполнении и его подтехне.
Пример вывода показан ниже:
===========================================================================================================================================
NNAPI timing summary (total time, ms wall-clock) Execution
----------------------------------------------------
Initialization Preparation Compilation I/O Compute Results Ex. total Termination Total
-------------- ----------- ----------- ----------- ------------ ----------- ----------- ----------- ----------
Application n/a 19.06 1789.25 n/a n/a 6.70 21.37 n/a 1831.17*
Runtime - 18.60 1787.48 2.93 11.37 0.12 14.42 1.32 1821.81
IPC 1.77 - 1781.36 0.02 8.86 - 8.88 - 1792.01
Driver 1.04 - 1779.21 n/a n/a n/a 7.70 - 1787.95
Total 1.77* 19.06* 1789.25* 2.93* 11.74* 6.70* 21.37* 1.32* 1831.17*
===========================================================================================================================================
* This total ignores missing (n/a) values and thus is not necessarily consistent with the rest of the numbers
Сигнал может потерпеть неудачу, если собранные события не представляют полного следа приложения. В частности, это может потерпеть неудачу, если в Systrace события, сгенерированные для обозначения конца раздела, присутствуют в трассировке без связанного события начала секции. Обычно это происходит, если при запуске Systrace Collector генерируются некоторые события из предыдущего сеанса профилирования. В этом случае вам придется снова запустить свое профилирование.
Добавить статистику для кода приложения в Systrace_parser
Приложение Parse_systrace основано на встроенной функциональности Android Systrace. Вы можете добавить следы для конкретных операций в вашем приложении, используя Systrace API ( для Java , для нативных приложений ) с помощью пользовательских имен событий.
Чтобы связать свои пользовательские события с этапами жизненного цикла приложения, приготовьте название вашего события с одной из следующих строк:
-
[NN_LA_PI]
: событие уровня приложения для инициализации -
[NN_LA_PP]
: событие уровня приложения для подготовки -
[NN_LA_PC]
: событие уровня приложения для компиляции -
[NN_LA_PE]
: событие уровня приложения для выполнения
Вот пример того, как вы можете изменить пример примера классификации изображений TFLITE, добавив раздел runInferenceModel
для фазы Execution
и слой Application
, содержащий другие другие разделы preprocessBitmap
, который не будет рассматриваться в трассах NNAPI. Раздел runInferenceModel
будет частью событий Systrace, обработанных синестровским анализатором NNAPI:
Котлин
/** Runs inference and returns the classification results. */ fun recognizeImage(bitmap: Bitmap): List{ // This section won’t appear in the NNAPI systrace analysis Trace.beginSection("preprocessBitmap") convertBitmapToByteBuffer(bitmap) Trace.endSection() // Run the inference call. // Add this method in to NNAPI systrace analysis. Trace.beginSection("[NN_LA_PE]runInferenceModel") long startTime = SystemClock.uptimeMillis() runInference() long endTime = SystemClock.uptimeMillis() Trace.endSection() ... return recognitions }
Ява
/** Runs inference and returns the classification results. */ public ListrecognizeImage(final Bitmap bitmap) { // This section won’t appear in the NNAPI systrace analysis Trace.beginSection("preprocessBitmap"); convertBitmapToByteBuffer(bitmap); Trace.endSection(); // Run the inference call. // Add this method in to NNAPI systrace analysis. Trace.beginSection("[NN_LA_PE]runInferenceModel"); long startTime = SystemClock.uptimeMillis(); runInference(); long endTime = SystemClock.uptimeMillis(); Trace.endSection(); ... Trace.endSection(); return recognitions; }
Качество обслуживания
В Android 11 и выше NNAPI обеспечивает лучшее качество обслуживания (QOS), позволяя приложению указать относительные приоритеты своих моделей, максимальное количество времени, которое, как ожидается, подготовит данную модель, и максимальное количество времени, которое, как ожидается, завершит заданное вычисление. Android 11 также вводит дополнительные коды результатов NNAPI, которые позволяют приложениям понимать сбои, такие как сроки пропущенного выполнения.
Установите приоритет рабочей нагрузки
Чтобы установить приоритет рабочей нагрузки NNAPI, вызовите ANeuralNetworksCompilation_setPriority()
до вызова ANeuralNetworksCompilation_finish()
.
Установить сроки
Приложения могут устанавливать сроки как для компиляции модели, так и для вывода.
- Чтобы установить тайм -аут компиляции, вызовите
ANeuralNetworksCompilation_setTimeout()
, прежде чем вызоватьANeuralNetworksCompilation_finish()
. - Чтобы установить тайм -аут вывода, вызовите
ANeuralNetworksExecution_setTimeout()
до запуска компиляции .
Подробнее о операнде
В следующем разделе рассматриваются расширенные темы об использовании операндов.
Квантовые тензоры
Квантовый тензор-это компактный способ представления N-размерной массивы значений плавающей запятой.
NNAPI поддерживает 8-битные асимметричные квантовые тензоры. Для этих тензоров значение каждой ячейки представлено 8-битным целым числом. С тензором связан масштаб и нулевое значение. Они используются для преобразования 8-битных целых чисел в значения плавающей точки, которые представлены.
Формула:
(cellValue - zeroPoint) * scale
где значение нулевого значения является 32-разрядным целым числом, а шкала-32-разрядное значение плавающей запятой.
По сравнению с тензорами 32-битных значений плавающей запятой, 8-битные квантовые тензоры имеют два преимущества:
- Ваше приложение меньше, так как обученные веса занимают четверть от 32-битных тензоров.
- Вычисления часто можно выполнять быстрее. Это связано с меньшим количеством данных, которые необходимо извлечь из памяти и эффективность процессоров, таких как DSP, при выполнении целочисленной математики.
Хотя можно преобразовать модель с плавающей запятой в квантованную, наш опыт показал, что лучшие результаты достигаются путем непосредственного обучения квантовой модели. По сути, нейронная сеть учится компенсировать повышенную гранулярность каждого значения. Для каждого квантового тензора значения масштаба и нулевых значений определяются во время учебного процесса.
В NNAPI вы определяете квантованные типы тензоров, устанавливая поле типа ANeuralNetworksOperandType
структуры данных ANEURALNETWORKS_TENSOR_QUANT8_ASYMM
. Вы также указываете масштаб и нулевое значение тензора в этой структуре данных.
В дополнение к 8-битным асимметричным квантовым тензорам NNAPI поддерживает следующее:
-
ANEURALNETWORKS_TENSOR_QUANT8_SYMM_PER_CHANNEL
, который вы можете использовать для представления весов для операцийCONV/DEPTHWISE_CONV/TRANSPOSED_CONV
. -
ANEURALNETWORKS_TENSOR_QUANT16_ASYMM
, который вы можете использовать для внутреннего состоянияQUANTIZED_16BIT_LSTM
. -
ANEURALNETWORKS_TENSOR_QUANT8_SYMM
, который может быть вводом дляANEURALNETWORKS_DEQUANTIZE
.
Дополнительные операнды
Несколько операций, такие как ANEURALNETWORKS_LSH_PROJECTION
, принимают дополнительные операнды. Чтобы указать в модели, что необязательный операнд опущен, вызовите функцию ANeuralNetworksModel_setOperandValue()
, проходя NULL
для буфера и 0 для длины.
Если решение о том, присутствует ли операнд или нет, варьируется для каждого выполнения, вы указываете, что операнд пропущен, используя функции ANeuralNetworksExecution_setInput()
или ANeuralNetworksExecution_setOutput()
, проходя NULL
для буфера и 0 для длины.
Тенсоры неизвестного звания
Android 9 (API -уровень 28) представил модельные операнды неизвестных измерений, но известного ранга (количество измерений). Android 10 (уровень API 29) представил тензоры неизвестного ранга, как показано в AneurnetWorksOperAndType .
NNAPI
Бланка NNAPI доступен на AOSP в platform/test/mlts/benchmark
(Benchmark App) и platform/test/mlts/models
(модели и наборы данных).
Конфликт оценивает задержку и точность и сравнивает драйверы с той же работой, выполненной с использованием Tensorflow Lite, работающего на процессоре, для тех же моделей и наборов данных.
Чтобы использовать эталон, сделайте следующее:
Подключите целевое устройство Android к компьютеру, откройте окно терминала и убедитесь, что устройство достижимо через ADB.
Если подключено более одного устройства Android, экспортируйте целевое устройство
ANDROID_SERIAL
Environment Variable.Перейдите в справочный справочник Android Top Source.
Запустите следующие команды:
lunch aosp_arm-userdebug # Or aosp_arm64-userdebug if available ./test/mlts/benchmark/build_and_run_benchmark.sh
В конце эталонного запуска его результаты будут представлены в виде HTML-страницы, переданной в
xdg-open
.
NNAPI журналы
NNAPI генерирует полезную диагностическую информацию в системных журналах. Чтобы проанализировать журналы, используйте утилиту LogCat .
Включите многословную регистрацию NNAPI для конкретных фаз или компонентов, установив свой свойства debug.nn.vlog
(с использованием adb shell
) для следующего списка значений, разделенных пространством, толстой кишкой или запятой:
-
model
: построение модели -
compilation
: генерация плана выполнения модели и компиляции -
execution
: выполнение модели -
cpuexe
: выполнение операций с использованием реализации процессора NNAPI -
manager
: расширения NNAPI, доступные интерфейсы и информацию, связанные с возможностями -
all
или1
: все элементы выше
Например, для включения полного журнала словесного регистрации используйте команду adb shell setprop debug.nn.vlog all
. Чтобы отключить регистрацию словеса, используйте команду adb shell setprop debug.nn.vlog '""'
.
После включения, журнала Verbose генерирует записи журнала на уровне информации с тегом, установленным на фазу или имени компонента.
Помимо контролируемых сообщений debug.nn.vlog
, компоненты API NNAPI предоставляют другие записи журнала на разных уровнях, каждый из которых использует конкретный тег журнала.
Чтобы получить список компонентов, найдите дерево источника, используя следующее выражение:
grep -R 'define LOG_TAG' | awk -F '"' '{print $2}' | sort -u | egrep -v "Sample|FileTag|test"
Это выражение в настоящее время возвращает следующие теги:
- Burstbuilder
- Обратные вызовы
- Компиляция строителя
- CPUEXECUTOR
- CERSUTIONBUILDER
- CERSUTIONBURSTCONTROLLER
- CERSUTIONBURSTSERVER
- FEECTUTIONPLAN
- Фибонакцидривер
- График
- IndexedShapeWrapper
- Ionwatcher
- Менеджер
- Память
- Memoryutils
- Метамодель
- Modelargumentinfo
- Модельный задумчик
- Neuralnetworks
- Операция
- Операции
- Операции
- PackageInfo
- Tokenhasher
- TypeManager
- Утилит
- ValidateHal
- Версииэнтерфейсы
Чтобы управлять уровнем сообщений журнала, показанного logcat
, используйте переменную среды ANDROID_LOG_TAGS
.
Чтобы показать полный набор сообщений журнала NNAPI и отключить любые другие, установите ANDROID_LOG_TAGS
на следующее:
BurstBuilder:V Callbacks:V CompilationBuilder:V CpuExecutor:V ExecutionBuilder:V ExecutionBurstController:V ExecutionBurstServer:V ExecutionPlan:V FibonacciDriver:V GraphDump:V IndexedShapeWrapper:V IonWatcher:V Manager:V MemoryUtils:V Memory:V MetaModel:V ModelArgumentInfo:V ModelBuilder:V NeuralNetworks:V OperationResolver:V OperationsUtils:V Operations:V PackageInfo:V TokenHasher:V TypeManager:V Utils:V ValidateHal:V VersionedInterfaces:V *:S.
Вы можете установить ANDROID_LOG_TAGS
, используя следующую команду:
export ANDROID_LOG_TAGS=$(grep -R 'define LOG_TAG' | awk -F '"' '{ print $2 ":V" }' | sort -u | egrep -v "Sample|FileTag|test" | xargs echo -n; echo ' *:S')
Обратите внимание, что это всего лишь фильтр, который применяется к logcat
. Вам по -прежнему нужно установить свойство debug.nn.vlog
для all
, чтобы генерировать информацию журнала словеса.